
Introduction
Within NGFN-2, large-scale case-control studies for the identification of predisposing genes for complex diseases are planned. Many of the susceptibility genes, however, act through modification of disease risk associated with life-style and/or environmental factors. Thus statistical analysis of gene-environmental interactions is required for the investigation of the possible mechanisms involved.
While there are several important examples of interactions, the full extend of their relevance is not clear from available data. Moreover, the meaning of interaction varies between statistical and biological sciences, as precise definitions are often omitted. We argue that the investigation of gene-environment (G x E) interaction is mostly sensible in advanced stages of genetic research, for the detailed characterization of identified disease genes or the stratified analysis of environmental effects by genotype. The widespread use of GxE interaction for targeted intervention or personalized treatment (pharmacogenetics) is still beyond current means; hardly any such interaction is used in clinical practice due to unconvincing evidence or low predictive and discriminative power. Valid results on G x E interactions require studies that include large sample sizes, corrections for multiple testing and replication.
The objective of the project is to evaluate the relative benefits of different approaches for the statistical analysis of gene-environmental interactions based on single nucleotide polymorphisms and haplotypes using simulated data and to employ these methods to real data sets.
Project Status
Definition of interaction
One will often notice that both different connotations and different concepts of the term interaction are used by statisticians, clinicians, biologists, geneticists. Frequently, a precise definition is completely omitted, which may lead to some confusion and controversy between scientists of different disciplines. In a biological context, interaction usually means co-participation in the same causal mechanism of disease development. This may be considered as a direct reaction of a certain exposure with e.g. an enzyme whose detoxification ability depends on the genotype of a certain gene. For more complex situations, abstract approaches of defining biologic interaction have been developed such as the counterfactual and sufficient-cause definitions. In most cases however, the underlying biological complexity cannot be adequately represented by simplified models. Another problem with such a mechanistic definition is that quite different causal relationships can lead to the same pattern of observed data and thus cannot be distinguished from epidemiological data.
On the other hand is the definition of statistical interaction, which does not imply any inference about particular biological modes of action. Statistical interaction is usually defined as “departure from additivity of effects on a specific outcome scale” (1). In Genetic Epidemiology, a common effect measure is the genotype relative risk with homogeneity of effect corresponding to multiplicativity of the respective relative risks. Numerical examples are given in table 1 for the additive scale as well as for the multiplicative scale.
Note that whether two factors show a statistical interaction crucially depends on the chosen outcome scale. Moreover, if there is evidence for both G and E main effects and there is no interaction on a multiplicative risk ratio scale, this implies that there must be an interaction on an additive risk ratio scale. Alternatively, statistical interaction can also be viewed as effect measure modification, i.e. the presence or absence of one risk factor modifies the effect measure, e.g. risk ratio, of a second risk factor. Depending on the study purpose this is also described as heterogeneity of effects in strata. The interpretation of which factor modifies the effect of the other depends on the design and objective of the study.
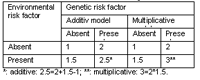
Study design
In general, almost all study designs used in genetic epidemiology can be extended to investigate G x E interaction and specific methods have been proposed for their analysis. Linkage studies aim at identifying genomic regions that are physically close to the disease gene - regions which are shared identical by descent between affected family members. Specific methods for G x E interaction in linkage studies have been developed e.g. for the affected sib-pair design and for linkage studies of quantitative traits. Studies that explore associations between a disease and genotypes are a second widely used approach to gene identification. Here, the number of genotypes investigated can vary greatly, from one functional polymorphism in a candidate gene to around a hundred thousand SNPs in genome-wide association studies. Family based studies, e.g. case-parent or sibling studies, and population based case-control studies can be used to analyze G x E interaction. If the interest is only in the G x E interaction, the special “case-only” design exists. The idea of this design is based on the assumption that genotype and environmental exposure are independent in the base population, so that exposure should be equal among subgroups defined by genotype. The case-only design was shown to be more efficient than the traditional case-control design (see figure 1), but since the assumption of independence is not assessable in the case sample alone, the design is prone to bias and confounding.
Power and sample size
The sample size required to detect a statistically significant G x E interaction is generally larger than the sample size to identify a G or E main effect. Figure 1 shows the required sample size for association studies in a candidate gene approach for three different study designs. The desirable large sample sizes are not always achievable, e.g. because of difficult or time-consuming phenotyping, limited availability of the required biological material (analysis tissue samples or DNA adducts) or financial constraints. Therefore, smaller initial studies will often be performed which are important and valid first steps in research. But they should be considered rather exploratory, and the emphasis in reporting should not be so much on the statistical significance but rather on confidence intervals of effect estimates and whether the observed effects could be of a clinically relevant size. Additionally the biological plausibility of the observed interaction should be critically discussed and potential confounders or intermediate pathways explored. Such smaller studies can generate valuable hypotheses which should then be definitely confirmed or refuted in larger studies.
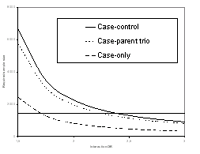
Multiple tests
In general, a common shortcoming of studies claiming a G x E interaction is that no plausible a priori hypotheses are defined. Statistical tests of interactions are conducted between all available genetic and environmental variables, sometimes even in several subgroups of the data, without adequate correction for multiple testing and more stringent significance levels leading to many false positive results. The inclusion and testing of interactions greatly increases the number of statistical tests and thus the need to correct for multiple testing. This might lower power to identify a relevant gene. Figure 2 shows the increase of the Family Wise Error Rate (FWER) depending on the number of hypotheses. The FWER denotes the probability of having at least one falsely significant test result within the set of tested hypotheses.
Outlook
Validation of statistical methods for testing GxE interaction using SNPs and haplotypes
Advanced statistical methods will be implemented for the analysis of candidate genes in complex disease. Several classes of methods were recently proposed to assess statistical gene-environment interaction, and evaluated in specific situations. The methods vary in sample design, the type of genetic information as well as in the types of the response variables and the possibility to adjust for additional covariates. The aim of the project is to establish these methods, to analyse them in realistic situations, and to give recommendations about the use of the methods with focus on the genetic data. Power comparisons between the methods are restricted because of different preconditions of study design and the types of non-genetic and genetic data. For interested scientists, we plan to present a detailed overview of the methods in the internet. The presentation will include the study design, type of data and outcomes for which the methods are proposed as well as links to the software packages and additional resources.
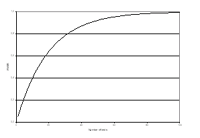
Simulation
Exhaustive simulation studies are required for the evaluation of statistical methods for haplotype estimation and for subsequent association analyses. We will use the SNAP software, which is developed in the SMP-GEM project on “Haplotypes and genotypes in association studies”. SNAP can generate genotypic data for simulated case-control and nuclear family samples under disease models. Environmental effects will also be implemented. The simulation tool offers a unified framework for simulating appropriate datasets that resemble complex diseases, and will be used to evaluate and to compare different statistical methods for the analysis of gene-environment interaction, including haplotype estimation.
Haplotype analysis
The performance of haplotype-based methods relies on a reliable estimation of the haplotypes. Haplotype reconstruction methods are sensitive to low frequency haplotypes in the range of 1% to 10%, due to sampling error and methodological issues. Genotyping errors and the imputation of missing data also increase the complexity of haplotype reconstruction. In cooperation with the project “Haplotypes and genotypes in association studies”, we will analyze different approaches to haplotype estimation and subsequent association analysis with respect to: (1) accuracy of haplotype estimation, and (2) the validity and power of the association analysis.
Lit.: 1. Rothman and Greenland Modern Epidemiolog, 2nd edt Lippincott-Raven, Philadelphia, 1998. 2. Gauderman WJ Sample Size requirements for matched case-control stuides of gene-environment interaction. 2002 Stat Med 21:35-50.