Responsible: Dr. Andreas Ruepp, GSF, Munich.
Introduction
The availability of whole genome sequences for an increasing number of metazoan species greatly enhances our understanding of the function of human genes by comparative genome analysis. The detailed characterization of the structure and variation of individual genes, functional motifs in protein sequences and non-coding sequences in the human population is in most cases not sufficient to relate genes to phenotypes. The key challenge in the future will be to analyse the various interactions of regulatory DNA, RNAs, proteins and metabolites to understand the functionalities of regulatory and signaling networks at higher levels, including homeostatic and pleiotropic responses to pharmacological or environmental perturbations.
No systematic comprehensive analysis of the functional dependencies amongst genes and their correlation to expression profiles has yet been achieved. The aim of this subproject is therefore to use information generated in independent experiments to explore the correlation of known interactions to expression profiles. Any set of genes can be represented as a network consisting of interconnected subnets or clusters equivalent to functional modules. The subdivision of any set of genes into modules reduces the complexity of possible and plausible interactions substantially, allowing for a more rational interpretation of experimental data (Dietmann et al., 2005). It is evident that modularization is a straightforward and highly successful basic biological concept. Interaction networks are logically but not mechanistically linked to transcript regulation. Identification of disturbed interactions with respect to transcription control (e.g. uncoupling of cross-correlating, co-regulated genes) in samples from the clinical networks may provide a rational interpretation of the disease mechanisms (e.g. rheumatic arthritis, infection and inflammation).
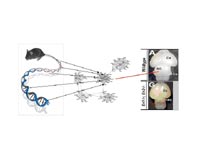
Planned work:
-
GenSet Collection: Diverse datasets will be generated from functional classification, regulatory information, metabolic and regulatory pathways, protein/protein interactions and comparative approaches. Application of clustering algorithms will return a set of putative GenSets representing functional modules.
-
Mapping algorithms: Given the graph of candidate clusters as generated in (1), high-throughput data will be systematically screened for cross-correlation of co-expressed genes with genes linked within the cluster candidates. Vice versa, sets of co-expressed genes will be mapped to candidate clusters.
-
Optimization of parameters: For any gene, a functional neighborhood can be described by a set of relations. To quantify any possible relations, a quantitative description of the functional distance is required. Since many of the values are missing (unknown relations), the gene sets will be dynamic. Optimization by updating gene sets as well as optimization of parameters.
-
Comparision of independent experiments: Here we aim to scan public as well as NGFN array data to correlate detected patterns to GeneSets. These patterns will be linked to the experimental condition, e.g. tumor type. Thus, beyond the use of signals as markers for disease states, additional information will be available for the interpretation of the differences between disease and homöostatic conditions.
-
Confirmation of module dependencies: Based on our bioinformatic analysis, we will interact with experimentalists to test hypothetical functional assignments based on our GenSet approach. In particular, within the SMP model organisms, the functional analysis of mouse mutants offers a sensible approach for the confirmation of hypotheses derived from data analysis, by reducing the experimental space significantly to a relatively small number of testable conditions.
Project Status
GeneSet collection
Groups of genes that display correlations in different networks have a high probability of being functionally correlated and therefore represent functional modules. In order to derive the Genset collection, different classes of experimental (BIND, http://bind.ca; MIPS Mammalian protein interaction database, Pagel et al., 2005) and predicted (DIMA, Pagel et al., 2004) data sets on interactions and co-expression networks networks (SymAtlas) were integrated for both human and mouse cellular networks. Probabilistic networks were calculated by comparing the networks with functional annotation schemes and pathway repositories, such as FunCat, KEGG and GO. For example, Rhodes et al. recently performed a probabilistic analysis to derive a catalogue of all human protein-protein interactions integrating interaction and co-expression data of orthologous genes from model organisms such as fly, worm and yeast.
However, a systematic determination or analysis of functional modules, their dependencies and their correlation with expression profiles in man or mouse has not yet been performed. Integrating interaction data from different experimental sources, as well as transferring related data from other model organisms is necessary to derive a comprehensive catalogue of functional associations between any group of genes. Other information such as the presence of common promoter binding sites in upstream regions (e.g. from the CORG database) and literature co-citation networks will be integrated.
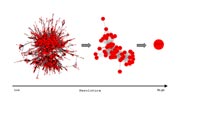
GenSets will be released in the GenRE annotation management system developed at MIPS (V. Stuempflen). that integrates interaction information for different model organisms. These data will be accessed by EJB’s including tested clustering routines with appropriate parameters.
Optimiziation of parameters
To assess the probability of functional association and the contribution from different sources of interaction information for any groups of genes, we apply heterogeneous hidden markov models. Functional modules are derived by advanced clustering methods, such as SPC-Clustering (Tornow and Mewes, 2003).
Outlook
The focus of the project will be to interact with experimentalists for confirming computationally derived functional modules, for relating them to disease phenotypes and for testing hypothetical functional assignments based on our GeneSet approach. Within the SMP model organisms, the functional analysis of mouse mutants (M. Hrabe de Angelis) or, in particular, the analysis of gene networks involved in adipositas (G. Brockmann) offer a sensible approach for the confirmation of hypotheses derived from data analysis.
Lit.: 1. Dietmann S. et al. Resources and tools for investigating biomolecular networks in mammals. Curr Pharm Des. 2005 (in press) 2. Pagel P. et al. The MIPS mammalian protein-protein interaction database Bioinformatics. 2005 Mar;21(6):832-4. 3. Pagel P. et al. A domain interaction map based on phylogenetic profiling. J Mol Biol. 2004 Dec 10;344(5):1331-46. 4. Ruepp A. et al. The FunCat, a functional annotation scheme for systematic classification of proteins from whole genomes. Nucleic Acids Res 32(18):5539-45, 2004. 5. Rhodes DR. et al. Probabilistic model of the human protein-protein interaction network. Nat Biotechnol 2005; 23(8):951-959. 6. Townow S. and Mewes HW. Functional modules by relating protein interaction networks and gene expression. Nucleic Acids Res. 2003 Nov 1;31(21):6283-9.