
Introduction
The mere number of 20.000 to 30.000 protein-coding genes in mammalian species is not sufficient to explain the complexity of the mammalian Bauplan, its development during ontogenesis, its physiology and its highly integrated response to biotic and abiotic environmental factors. Instead, molecular complexity is dramatically increased at the protein level and through the spatial and temporal regulation of gene expression. Thus coordinated gene regulation is integral to pleiotropic gene functions. In this context research in the Molecular Phenotyping Screen focuses on the analysis of gene regulation in mouse models for human diseases primarily at the transcript level. In the German Mouse Clinic (GMC) we established genome-wide expression studies using the DNA-chip technology complementary to ‘classical’ phenotyping screens (1).
Results/Project Status
Setting up the DNA-chip plattform
Within the German Mouse Clinic (GMC) we are using our DNA-Chip technology to molecularly phenotype mouse mutant lines on a large-scale and high-throughput basis (2). A panel of 14 distinct organs from male mutant and wild-type mice at the age of 105 (±5) days are routinely archived from every mouse line that is examined in the GMC. Optionally additional organs of male and female mice at other ages are analysed on request. So far, more than 7100 organs from 39 mutant and 5 wild-type strains have been archived. Sample selection for DNA-chip expression profiling is based on conspicuous phenotypes in screens of the GMC. 43 different organs of 22 mutant mouse lines were selected for molecular phenotyping in up to 20 chip hybridisations per organ and completely analysed. In 13 mutant lines candidate genes for differential gene expression have been identified in 25 different organs. ombination of classical phenotyping and expression profiling is an efficient tool to link phenotypes to underlying changes in gene expressions.
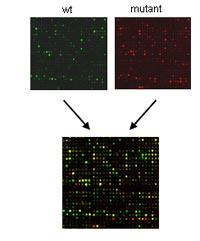
Assessment of DNA-chip specificity
The quality of DNA chip expression profiling data critically depends on the specificity of hybridisation data (3). We developed SAFE (Specificity Assessment from Fractionation Experiments), a novel method to discriminate between unspecific cross-hybridisation and specific signals (4). For this method we applied in situ fractionation of hybridised target on DNA-chips by means of repeated washes with increasing stringencies. Different fractions of hybridised target are washed off at defined stringencies and the collected fluorescent intensity data at each step comprise the fractionation curve. Based on characteristic features of the fractionation curve, unreliable data can be filtered and eliminated from subsequent analyses. This approach provides an experimental tool to identify probes that produce specific hybridisation signals in DNA-chip expression profiling approaches. Using SAFE we estimated the number of probes with unspecific cross-hybridization below 2%.
Assessment of a systematic expression profiling approach in mice
The systematic analysis of RNA expression data of mouse mutants supports the understanding of the underlying molecular biology in such mutants and provides new insights into mammalian gene function. In a direct comparison of DNA microarray experiments of individual versus pooled RNA samples of organs from mouse models we showed that individual RNA samples outperform pools in several aspects. Genes with high biological variability in their expression levels (noisy genes) were identified as false positives in pooled samples (5). We provided evidence that highly stringent housing conditions and standardised procedures for the isolation of organs significantly reduced biological variability in gene expression profiling experiments. Data on wild-type individuals demonstrated the positive effect of controlling variables such as social status, food intake before organ sampling, and stress with regard to reproducibility of gene expression patterns. Analyses of several organs from various mutant lines show in general low numbers of differentially expressed genes and we demonstrate the feasibility to detect transcriptionally affected organs employing RNA expression profiling as a tool for molecular phenotyping.
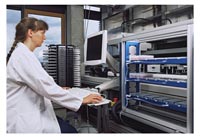
Gene expression profiling of disease models
As example, we have carried out a large scale RNA expression analysis of specific cell types commonly employed in studies of prion disease (6). Neuroblastoma cells (N2a) and hypothalamic neuronal cells (GT1) can be persistently infected with mouse adapted scrapie prions, the latter demonstrating cytopathologic effects associated with prion neuropathology. Using our DNA chip platform, we have identified several hundred differentially expressed sequences in the two cell lines when infected with prion strain RML. ScN2a and ScGT1 cells demonstrate unique changes in RNA profiles and both differ from the reported changes in human microglia and prion infected brain studies albeit with some overlap. In addition, several of the identified changes are shared in common with other neurodegenerative diseases such as Alzheimer's disease (AD). The results illustrate that prion infection differs in effect depending on the cell type which could be exploited for diagnostic or therapeutic intervention.
As a second example, we used our DNA-chip platform to detect ERBB2-regulated target genes that contribute to its tumorigenic effect on a genomewide scale (7). V-erb-b2 erythroblastic leukemia viral oncogene homolog 2 (ERBB2) encodes a transmembrane glycoprotein with tyrosine kinase-specific activity that acts as a major switch in different signal-transduction processes. ERBB2 amplification and overexpression have been found in a number of human cancers, including breast, ovary and kidney carcinoma. The differential gene expression profile of ERBB2-transfected and wild-type mouse fibroblasts was monitored employing DNA microarrays. Regulated expression of selected genes was verified by RT-PCR and validated by Western blot analysis. Genome wide gene expression profiling identified (i) known targets of ERBB2 signaling, (ii) genes implicated in tumorigenesis but so far not associated with ERBB2 signaling as well as (iii) genes not yet associated with oncogenic transformation, including novel genes without functional annotation. We also found that at least a fraction of coexpressed genes are closely linked on the genome. ERBB2 overexpression suppresses the transcription of antiangiogenic factors (e.g., Sparc, Timp3, Serpinf1) but induces expression of angiogenic factors (e.g., Klf5, Tnfaip2, Sema3c). Profiling of ERBB2-dependent gene regulation revealed a compendium of potential diagnostic markers and putative therapeutic targets. Identification of coexpressed genes that colocalize in the genome may indicate gene regulatory mechanisms that require further study to evaluate functional coregulation.
Also, we used DNA-chip expression profiling and 2D-gel electrophoresis combined with peptide mass fingerprinting to identify genes and proteins differentially regulated in E10.5 Dll1 (delta-like 1, Delta1) mutant embryos (8). The Notch signaling pathway has pleiotropic functions during mammalian embryogenesis. It is required for the patterning and differentiation of the presomitic and somitic paraxial mesoderm and of the neural tube. The differential expression profiling approach identified 47 regulated transcripts and 40 differentially expressed proteins. The majority of these genes has until now not been associated with Notch signaling. Subsequent whole-mount in situ hybridization confirmed that a subset of the identified transcripts has restricted and distinct patterns of expression in E10.5 mouse embryos. For most genes these expression patterns were affected in the presomitic mesoderm, in differentiating somites of Dll1 mutant embryos and in the neural tube and cells differentiating from it. Similar effects were observed in embryos homozygous for the Headturner (Htu) and pudgy (pu) mutations, which are alleles of the Notch ligands Jag1 and Dll3. The regulated expression of a subset of the proteins was validated by immunoblots. Remarkably six of the proteins down-regulated in Dll1 mutant embryos are proteasome subunits. The large set of regulated genes identified in this differential expression profiling approach is an important resource for further functional studies.
Outlook
The standardized phenotypic analysis of mouse models for human diseases is a fundamental requirement for the efficient and reliable analysis of mouse mutants (9). Within the German Mouse Clinic we generate transcript profiles of disease models using the DNA-chip technology complementary to ‘classical’ phenotyping screens. The analysis of such expression profiles will reveal target genes directly or indirectly regulated by a genetic modification, affected molecular pathways, groups of co-expressed genes, and potential targets for therapy. Organs of male mutant mice are systematically archived for subsequent RNA expression profiling analysis. Based on information from the GMC phenotyping screens organs to be analysed by DNA-chip analysis are specifically chosen for each mutant line. This strategy of combining systematic organ sampling and selected expression analyses has proven particularly efficient in the identification of differentially expressed genes in the frist phase of the GMC. We plan to continue this approach as an integral screen within the GMC. In addition, we are currently extending our custom made DNA-chip with new probes and we are employing recent in silico tools to identify affected pathways based on standardized Gene Ontology annotations.
Lit.: 1. J. Beckers, et al. Current Genomics 2002; 3, 121. 2. V. Gailus-Durner et al., Nat Methods. Jun, 2005; 2, 403. 3. A. L. Drobyshev et al., Current Genomics. 2003; 4, 615. 4. A. L. Drobyshev et al., Nucleic Acids Res. Jan 15, 2003; 31, E1. 5. M. Seltmann et al., Mamm Genome. Jan, 2005; 16, 1. 6: A. D. Greenwood et al., J Mol Biol. Jun 10, 2005; 349, 487. 7. J. Beckers et al., Int J Cancer. Apr 20, 2005; 114, 590. 8. C. Machka et al., Gene Expr Patterns. Jun 22, 2005. 9. J. Beckers and M. Hrabe de Angelis, Curr Opin Chem Biol. Feb, 2002; 6, 17.